Explore the benefits of AI-augmented software development with LLMs, including faster code generation, improved testing, and enhanced code quality.
In the rapidly evolving realm of software engineering, the integration of large language models (LLMs) is reshaping how businesses approach software development. Central to unlocking the full potential of LLMs is the fundamental skill of prompt engineering At its core, prompt engineering involves crafting input queries to extract precise and meaningful responses from LLMs. This pivotal skill empowers developers to elevate the accuracy and relevance of outputs, thereby optimizing the performance of AI applications.
Erik Meijer, an engineering director at Facebook, compares the emergence of LLMs to the transition from manual labor to utilizing heavy machinery. This analogy highlights the huge boost in efficiency and potential LLMs can bring to the software development processes. With the introduction of AI-powered tools, such as GitHub Copilot and Duet AI, developers can understand and write complex code, generate test cases, design and publish APIs, identify and fix errors, and write code documentation. These features enhance developers’ productivity and allow them to focus on creative aspects of software development.
For instance, developers at Turing, an AI-powered tech services company, experienced a remarkable 30% boost in productivity through the use of Duet AI. Another study highlighted a substantial improvement in task completion speed, revealing that developers leveraging GitHub Copilot finished tasks 55% faster than their counterparts without the tool.
LLMs and prompt engineering form a powerful duo, where precise prompts guide LLMs to deliver contextually relevant and informed outputs that transform software engineering tasks. Let’s explore how these innovative AI engineering tools, powered by LLMs, are shaping the landscape for AI engineers by offering efficiency and effectiveness in the ever-evolving world of artificial intelligence.
Let’s dive in!
Prompts and software engineering
The ability of LLMs to build upon a given prompt and produce context-appropriate outputs makes them exceptionally useful across applications. Unlike traditional models that require large amounts of manually labeled data, LLMs using prompt engineering can produce informed outputs from simple instructions.
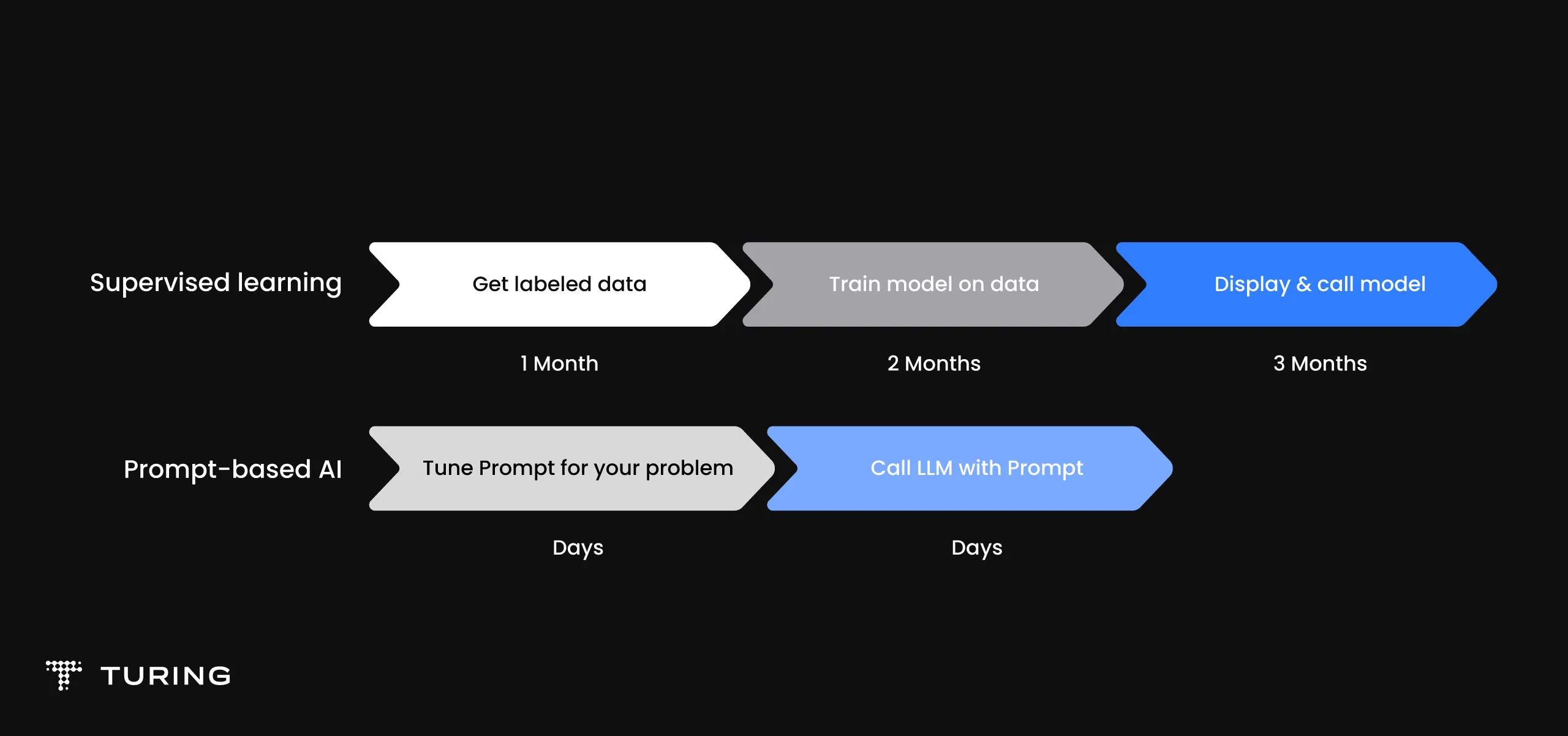
Prompts and software engineering
However, this innovation relies on creating and refining prompts to achieve the desired results.
As a developer you must learn to write effective prompts to build, test, deploy, and manage complex software solutions. This skill will enhance your ability to leverage AI tools to their full potential for streamlining workflows and improving the quality of the final product.
Here are a few tips to enhance the effectiveness of software engineering prompts:
- Be specific
Aim for clarity in your requests. The more detailed you are, the better the AI’s output.
Example:
Bad prompt: “Write some Python code for CSV files.”
Good prompt: “Create a Python function to read CSV files and list column names.”
- Direct instructions
Tell the AI exactly what to do.
Example:
Bad prompt: “Explain web APIs.”
Good prompt: “Describe the difference between POST and GET methods in web APIs.”
- Use examples
Provide examples to help the AI understand your goals.
Example:
Bad prompt: “Write a SQL query.”
Good prompt: “Write a SQL query for employees with salaries above $50,000, similar to this query for ages over 30: SELECT * FROM employees WHERE age > 30;”
- Refine your prompts
Begin with broad queries and narrow them down based on AI outputs.
Example:
Bad prompt : “How can I make a web app secure?”
Good prompt : “List methods to protect a web app from SQL injections and XSS.”
Strategic approaches for maximizing AI impact in development
Unlocking the full potential of LLM tools requires a strategic approach. Let’s explore essential recommendations to harness AI effectively to ensure adaptability, customization, collaboration, and continuous learning.
- Adapt and innovate: Stay agile by continuously exploring and experimenting with the latest AI tools and technologies. This proactive approach guarantees the ability to leverage the most effective solutions that align with evolving project needs and industry trends
- Focus on customization: Tailor your solutions to the distinct challenges and goals of each project. By fine-tuning LLMs and integrating specialized tools, this customized approach yields significant benefits that enhance efficiency, improve accuracy, and foster innovation.
- Enhance collaborative efforts: DuetAI and similar tools underscore the significance of collaboration between AI technologies and human expertise. Look at AI tools as your partners in the development process to both enhance productivity and spark creativity.
Integrating LLMs in software engineering
Key areas where LLM integration accelerates software development include:
Concept and planning: LLMs enhance brainstorming, requirements collection, and project scoping, turning ideas into detailed plans.
Tools: ChatGPT, Bing Chat, Bard, Character.ai
Design and prototyping: LLMs shorten the design cycle, providing instant prototypes and visual presentations.
Tools: Midjourney, Stable Diffusion, RunwayML, Synthesia.
Code generation and completion: LLMs automate coding tasks, improving efficiency and productivity.
Tools: GitHub Copilot, CodiumAI, Tabnine, DuetAI.
Code analysis and optimization: LLMs transform code examination by suggesting enhancements for performance and scalability.
Tools: p0, DuetAI, CodeRabbit, CodeQL, PR-Agent.
Test coverage and software testing: Generative AI tools, powered by LLMs, revolutionize software testing by automating the creation of unit, functional, and security tests through natural language prompts, providing alerts for potential code vulnerabilities and enhancing overall software quality.
Tools: Snyk, p0, TestGen-LLM.
Software architecture and documentation: LLMs aid in software design and generate comprehensive documentation.
Tools: Claude, ChatGPT, Cursor IDE.
Programming language translation: LLMs modernize legacy systems by translating code efficiently.
Tools: Gemini, OpenAI Codex, CodeBERT.
Bad prompt : “How can I make a web app secure?”
Good prompt : “List methods to protect a web app from SQL injections and XSS.”
Let’s delve deeper into their influence on development and coding.
LLMs in development and coding
Code generation: Creating code snippets in different programming languages according to specific needs.
Code review and optimization: Examining code for potential enhancements, optimization possibilities, and adherence to coding standards.
Bug fixing: Detecting bugs in code snippets and proposing solutions.
Documentation generation: Automatically producing documentation for code bases, including comments and README files.
Code explanation: Simplifying complex code logic or documenting the functionality of code blocks.
Learning new technologies: Offering explanations, examples, and tutorials for new programming languages, frameworks, or libraries.
Despite their impressive capabilities, LLMs have limitations that developers should be aware of. These can include difficulties in understanding context, generating misinformation, and raising ethical concerns.
Below we have outlined some of the limitations faced by current LLMs in code generation tasks, along with corresponding workarounds for developers to overcome these challenges.
Context understanding
Limitation: Sometimes, Large LLMs may not fully understand the context of a coding task, leading to mistakes in the code they generate.
Workaround: Provide detailed prompts with clear examples and refine based on the initial outputs.
Language support
Limitation: Certain LLMs might not work well with some programming languages or might be better at others.
Workaround: Choose LLMs with language specialization. You can also explore multilanguage models or consider manual translation for unsupported languages.
Complexity and scale
Limitation: LLMs might struggle with big or very complicated projects because they have limits on what they can handle.
Workaround: Decompose tasks, use modular design principles, combine LLM-generated code with handcrafted code, and leverage external libraries.
Syntax and logic
Limitation: LLMs might create code with mistakes like typos or incorrect instructions, especially for complex tasks.
Workaround: Review and validate code manually, utilize linting tools, and consider pair programming for quality assurance.
Domain-specific knowledge
Limitation: LLMs might not know everything about specific topics like specialized techniques or industry rules.
Workaround: Domain-specific knowledge: LLMs might not know everything about specific topics like specialized techniques or industry rules.
Ethical concerns
Limitation: There could be worries about the fairness of the code produced by LLMs or how it might be used in the wrong way.
Workaround:Implement ethical guidelines and considerations when using LLMs, regularly assess for biases, and prioritize transparency and fairness in outputs.
AI tools in the software development life cycle
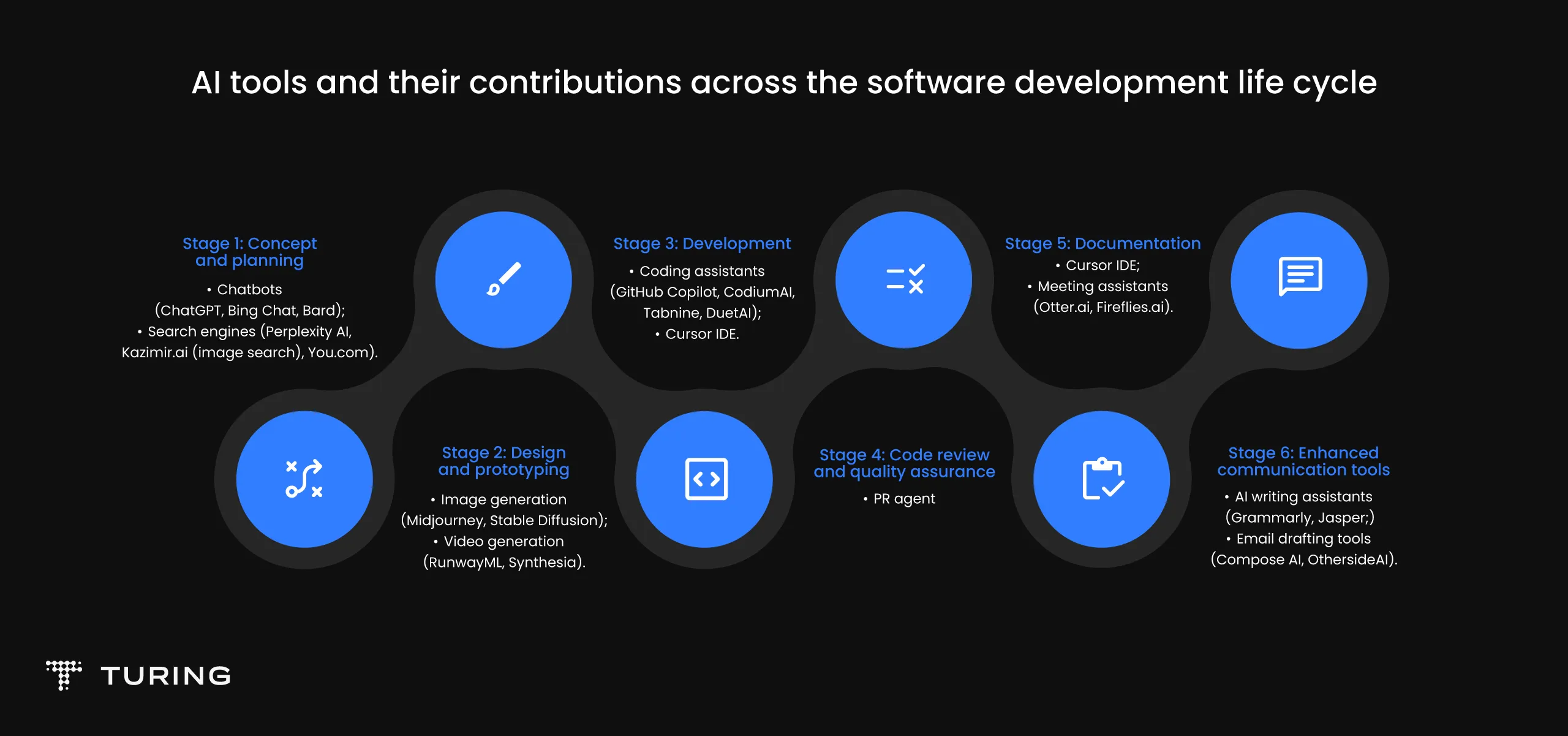
AI tools in the software development life cycle
From concept inception to deployment and maintenance, AI-driven solutions can streamline processes, enhance productivity, and drive innovation. Let’s explore the diverse array of AI tools and their impactful contributions across the software development life cycle.
Concept and planning
- Chatbots (ChatGPT, Bing Chat, Bard, Character.ai): Use for brainstorming, gathering requirements, and initial project scoping.
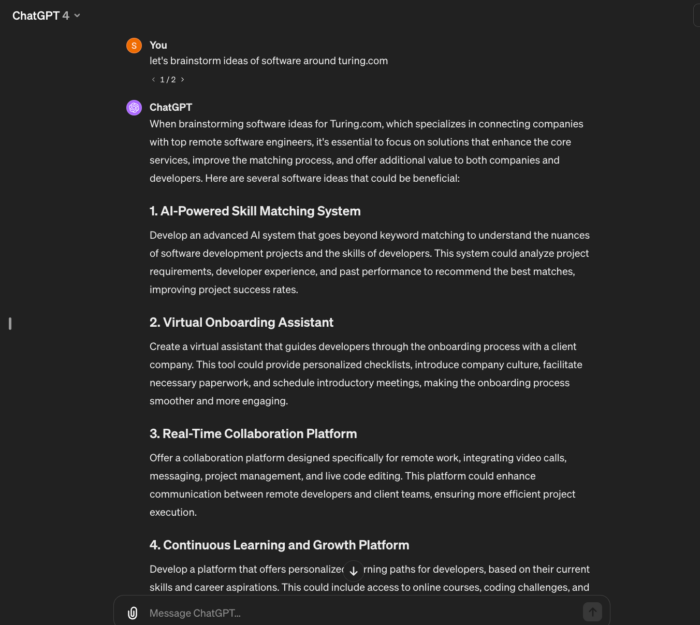
Source: Suraj Jadhav
- Search engines (Perplexity AI, kazimir.ai, You.com): Conduct preliminary research to validate ideas and explore existing solutions.
Design and prototyping
- Image generation (Midjourney, Stable Diffusion): Quickly create design mock-ups and UI/UX prototypes without extensive graphic design skills.
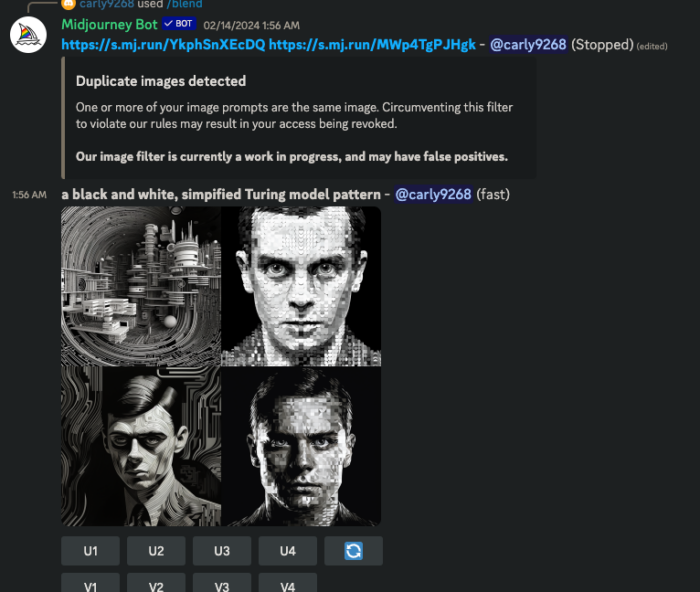
Source: Suraj Jadhav
- Video generation (RunwayML, Synthesia): Produce demo videos and visual presentations to communicate design concepts.
Development
- Coding assistants (GitHub Copilot, CodiumAI, Tabnine, DuetAI): Automate code writing, suggest optimal coding practices, and autocomplete codes.
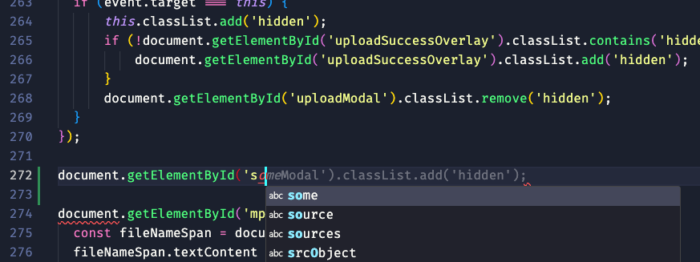
Source: Suraj Jadhav
- Cursor IDE: Integrated AI chat for real-time coding assistance that identifies potential bugs, suggests fixes, and creates documentation.
Code review and quality assurance
- PR agent: Automate code reviews to ensure adherence to best practices and identify potential issues.
Documentation
- Cursor IDE: Generate documentation by chatting with the code.
- Meeting assistants (Otter.ai, Fireflies.ai): Automatically transcribe meetings and generate documentation for team updates and decision logs.
Enhanced communication tools
- AI writing assistants (Grammarly, Jasper): Use these tools to ensure emails are clear, concise, and professionally written. They can help with grammar, tone, and style to make your communication more effective.
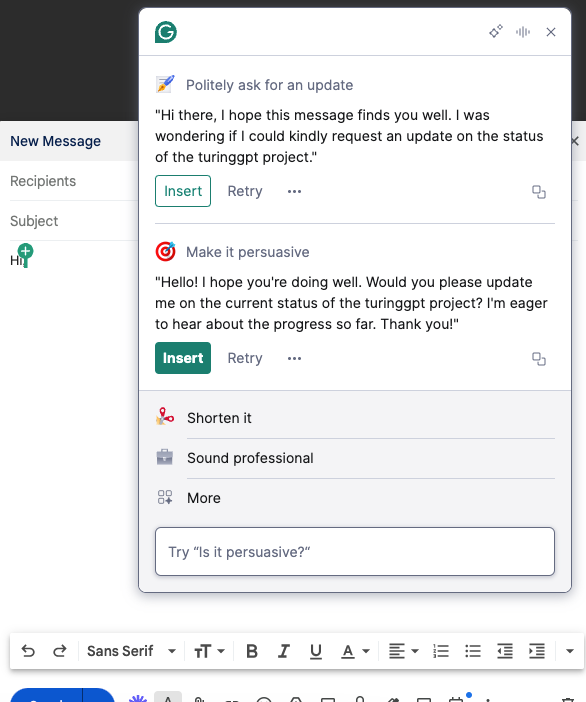
Source:Suraj Jadhav
- Email drafting tools (Compose AI, OthersideAI): Automate email drafting to save time and ensure consistency in communication. These tools can generate email content based on brief inputs, making it easier to maintain regular correspondence with stakeholders.
LLMs at Turing: Elevate your coding experience
The symbiosis between precise prompts and cutting-edge LLM tools has significantly elevated developer productivity, allowing developers to focus on creativity. Beyond prompt engineering, strategic approaches for maximizing AI impact underscore the importance of adaptation, customization, collaborative efforts, and a commitment to continuous learning.
- As an AI engineer, your role extends beyond acquiring knowledge of large language models; you’re a pivotal force in the world of LLMs. We invite you to be part of Turing’s LLM journey, where we promote healthy challenges, nurture growth, and empower our community to excel in the dynamic AI landscape. Apply now and be part of a community that thrives on innovation and exploration. Your journey with LLMs starts here!
FAQs
What are LLMs? Why are they used in software engineering?
LLMs are advanced AI tools designed to understand, generate, and work with humanlike language. Their integration into software development revolutionizes the way businesses build and manage applications by enhancing code generation, streamlining the software development life cycle, and enabling developers to focus more on creative problem-solving and less on repetitive coding tasks.
Are there any challenges associated with using LLMs in software engineering?
While LLMs offer tremendous benefits, their integration with software engineering processes comes with challenges. These include managing the accuracy of generated outputs, ensuring the AI’s solutions are contextually relevant, and addressing ethical considerations like data privacy and AI bias. Additionally, developers must be skilled in prompt engineering to communicate effectively with LLMs, and organizations must avoid overlooking the importance of human oversight.
How can developers leverage LLMs in their day-to-day work?
Developers can elevate their daily work by integrating LLMs into tasks like code generation, completion, analysis, and optimization. These models, equipped with advanced language understanding, significantly expedite software development processes by providing efficient solutions for various coding challenges.
What advancements are being made in the field of LLM research for software engineering?
Recent strides in LLM research for software engineering include refined prompt engineering techniques, improved code generation and completion capabilities, enhanced code analysis and optimization features, and the integration of LLMs in diverse stages of the software development life cycle.
Join a network of the world's best developers and get long-term remote software jobs with better compensation and career growth.
Apply for Jobs