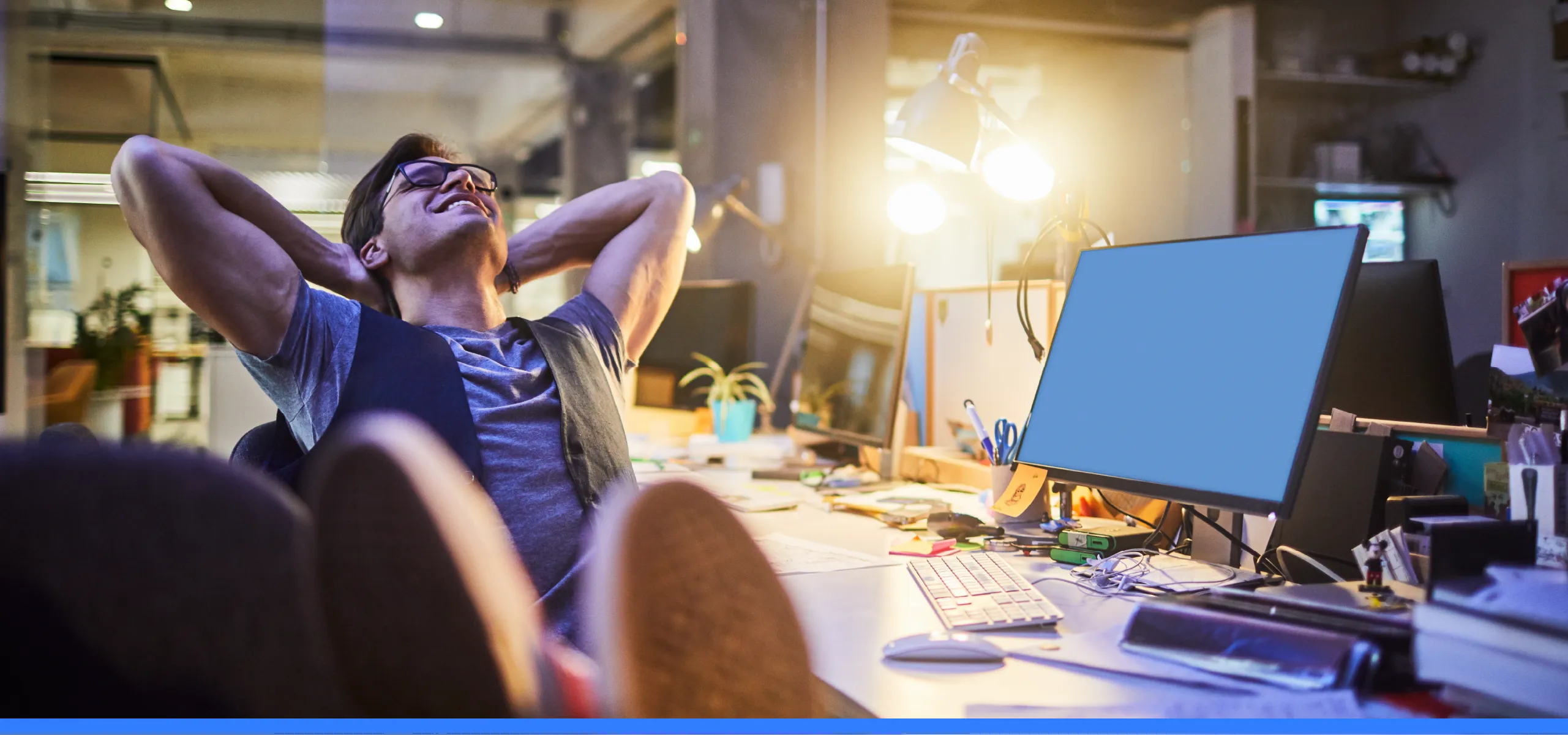
AI Myths Debunked: Why Your Job Is Safer than You Think
In the age of rapid technological advancement, and especially since the appearance of AI, one of the main concerns that have arisen is whether machines and algorithms will replace human jobs in the near future.
Media headlines often proclaim a looming era of mass unemployment, painting a dystopian picture where machines render human labor obsolete. But upon closer examination, many of these fears are unfounded. In fact, there are compelling reasons to believe that your job is likely safer than you think.
AI and the loss of human jobs: Three myths
1. Myth: AI will lead to widespread job loss across all industries.
Reality: While it’s true that automation can streamline certain tasks and processes, it’s important to recognize that AI is not a one-size-fits-all solution. Many jobs require a level of creativity, critical thinking, and emotional intelligence that machines simply cannot replicate.
Industries such as healthcare, education, and creative arts rely heavily on human expertise and empathy, making them less susceptible to automation.
2. Myth: A machine will be able to perform my daily tasks.
Reality: AI is often used to expand human capabilities rather than replace them entirely.
For example, in industries such as manufacturing and logistics, AI-powered robotics can enhance efficiency and productivity while releasing human workers from automatic tasks so they can focus on more complex tasks that require creativity.
By investing in education and training programs that equip workers with the skills needed to thrive in the digital economy, we can ensure that the benefits of AI are shared equitably across society.
Furthermore, history has shown that technological advancements often create new job opportunities. The rise of AI has led to the emergence of entirely new fields such as data science, machine learning engineering, and AI ethics.
As AI continues to evolve, so will the demand for skilled professionals who can apply its potential in innovative ways.
3. Myth: It’s easier and cheaper for companies to replace human workers with AI.
Reality: There is more to this assessment than whether an AI can perform your daily tasks or not—companies need to evaluate if it’s economically beneficial to have an AI replacing human workers.
Developing and deploying AI systems can require substantial investments in hardware, software, and training. Additionally, integrating AI into existing workflows and infrastructure can be complex and time-consuming, further adding to the initial cost of adoption.
How can I make my job safer?
As with any other groundbreaking advancement, jobs will suffer some modifications, and some of them might become obsolete. However, the implementation of AI will naturally create more job opportunities. Therefore, the best way to protect your current job is to get acquainted with AI and get the best out of it.
Moreover, the implementation of AI in the workplace presents some challenges. Concerns surrounding data privacy, algorithmic bias, and ethical implications have prompted organizations to approach AI adoption with caution. As a result, there is a growing demand for professionals who can ensure that AI systems are fair, transparent, and accountable.
Six new AI jobs
The following are some of the new job positions that have been emerging during the AI era:
AI product manager: Leads the development and launch of AI-powered products, defining product strategy, features, and requirements to meet customer needs and business goals
AI ethicist: Ensure the responsible and ethical development and deployment of AI technologies, addressing ethical considerations and societal impacts
Algorithm auditor: Verify the fairness, transparency, and compliance of AI algorithms with regulatory standards and ethical principles
NLP engineer: Specializes in natural language processing techniques to develop AI systems that understand and generate human language
Robotics engineer: Designs and builds robotic systems powered by AI to perform tasks autonomously, enhancing efficiency and productivity in various industries
AI research scientist: Conducts innovative research to advance the field of artificial intelligence, developing new algorithms and methodologies that solve complex problems
Even though they wouldn’t be considered new jobs, it’s also essential to highlight the contribution of machine learning engineers, data scientists, and computer vision engineers, among other professionals, who are currently playing pivotal roles in the advancement of AI technology.
Conclusion
In conclusion, while AI undoubtedly represents a significant technological advancement, the fear of widespread job loss is largely exaggerated. By debunking common myths and misconceptions surrounding AI, we can better understand its potential impact on the future of work.
Rather than viewing AI as a threat to employment, we should embrace it as a tool that can enhance human capabilities and drive economic growth. With the right approach, we can ensure that the jobs of tomorrow are not only safer but also more fulfilling and rewarding for workers around the world. If you are interested in this emerging technology, check our AI jobs opportunities.
Tell us the skills you need and we'll find the best developer for you in days, not weeks.