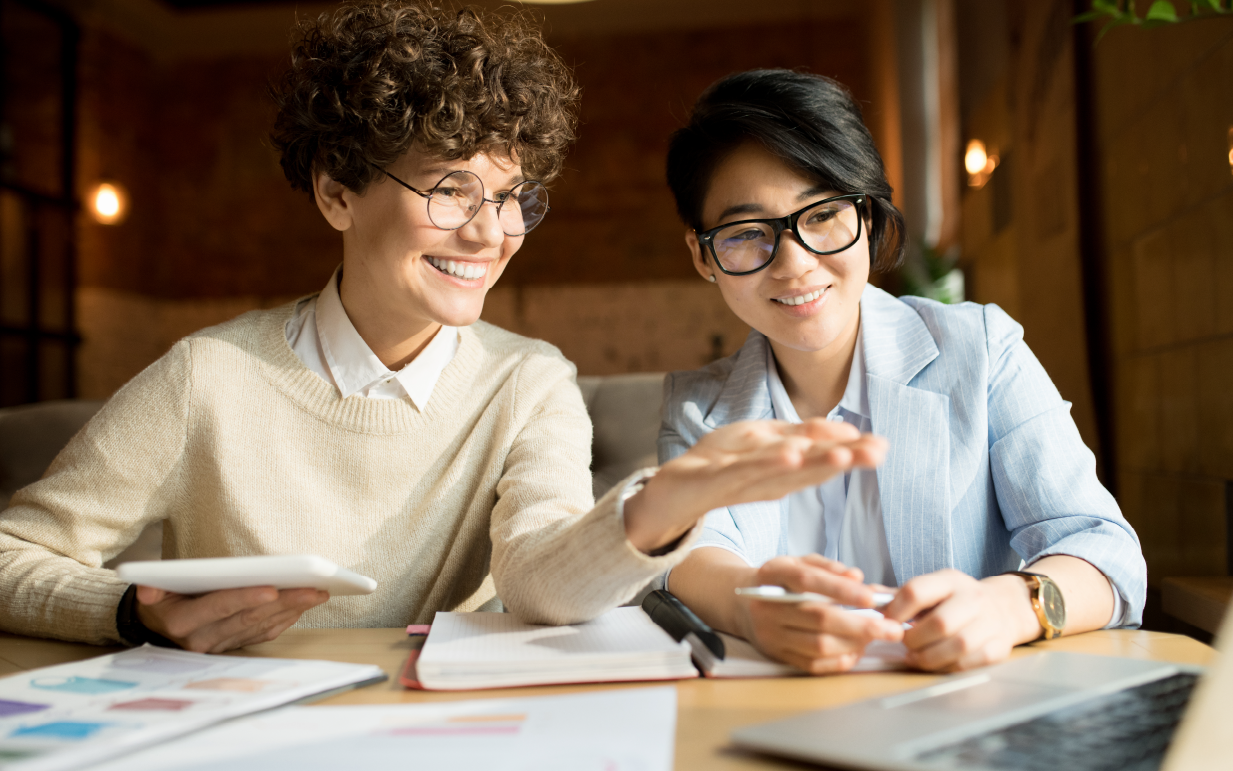
What is the Job of an EA in the AI Era?
Most AI tools are referred to as “assistants” and they’re intended to automate and facilitate daily tasks, especially the most repetitive ones. Tools like Calendly, Superhuman, Asana, and Slack help executives daily with calendar appointments, team collaboration, and email prioritization. Other AI tools like Siri, Google Assistant, or Alexa have conversational abilities and can help by making calls, reading texts, or setting up reminders. Sometimes, the support is so seamless that it leaves people wondering if AI will replace human assistants, like executive assistants (EAs).
The answer is: Probably not. As an executive, the best approach is to avoid extreme postures. Being afraid of new technologies can leave EAs without the proper toolkit to perform efficiently. On the other hand, assuming that AI can do the job of a top-tier executive assistant is far-fetched. It’s crucial to strike a balance between the value of AI and the value of human support.
How executive assistants are using AI
Photographers use all sorts of digital tools in their trade. Most use a digital camera and software like Photoshop and Lightroom. These tools help them do their job faster and even better, but they still need a great deal of technical expertise, an eye for detail, and training to produce the best images.
It’s a good example of how an executive assistant uses AI. EAs can use tools to record meetings and make transcripts or set automated replies or reminders for teams. They can leverage GenAI to build non-confidential decks or process playbooks. These are essential, useful tools. Yet, executive assistants need to show proactiveness, possess a high degree of intuition to make decisions, be ethical and trustworthy, and have ongoing training that will allow them to perform their duties efficiently.
The better we learn how to use AI tools and technology, the more benefit we’ll get from them.
EAs’ diverse tasks, responsibilities, and audiences require an assortment of skills and tools. LLMs and automation are greatly appreciated and empowering for executive assistants and are starting to become tools of the trade.
The following tasks are being documented and performed by the team of executive assistants at Viva.
Building usage databases for the entire team
The team of executive assistants built a centralized database with the collective knowledge of AI and LLMs’ usage. Every team member has access to edit and add new information about how LLMs have worked for them. The two top LLMs they use are ChatGPT (multiple versions) and Copilot (Bing).
The databases feature guides on usage like how to handle confidential information (and how to assess what is confidential), how to assess for ethical content, and how to confirm data. These guides also include prompt recommendations and basic structures for any team member’s use.
Here’s an example of a basic prompt structure found in said database:
Use cases of AIs and LLMs by executive assistants
Executive assistants use LLMs in a variety of ways. Here are some common EA tasks where LLMs prove useful:
Project management and vendor research
- Project management: Coordinate tasks and timelines, track progress, and ensure project objectives are met.
- [Prompt example] Summarize these notes into a bulleted list overview, and write all the action items in a separate list.
- [Prompt example] Create a detailed project plan for the [project name], including key milestones, deadlines, and task assignments. Ensure all tasks are clearly outlined and assigned to appropriate team members.
- Vendor research: Gather information on potential vendors, compare their offerings, and present options to the executive for decision-making.
- [Prompt example] Compare the offerings of the identified vendors, including pricing, services provided, and any unique features or benefits. Present the findings in a comparative table
- [Prompt follow-up] Gather customer reviews and testimonials for each vendor to assess their reliability and quality of service. Summarize the feedback and include it in the vendor comparison report.
Meeting and presentation support
- Create pre-meeting briefs: Summarize key points, agenda items, and background information for upcoming meetings to ensure the executive is well-prepared.
- [Prompt example] I’m meeting X for an {investor/sales/partnership/co-branding/etc} meeting. Create a pre-meeting brief about company XYZ (paste LinkedIn profile + webpage URL). Include these bullet points: the year it was founded, key information, industry, shared investors, business model, latest news, headquarters, fundraising stage, insights on anything else I’d need to know, and headcount.
- Create presentations (slide outline and content of non-confidential information): Develop slide decks with relevant content and visual aids to effectively convey information during presentations.
- [Prompt example] Ask ChatGPT to create a PowerPoint presentation and export it. Afterward, ask it to give you the VBA code. It will give you a code which you can then input in PowerPoint, and it will create the slides for you.
Writing and content creation
- Generate content ideas for social media: Brainstorm and propose engaging content ideas for social media platforms to enhance brand visibility and engagement.
- [Prompt example] “Brainstorm 10 engaging content ideas for our company’s LinkedIn page to enhance brand visibility and engagement in the tech industry.”
- [Prompt example] “Propose a week’s worth of Instagram posts for our lifestyle brand, focusing on promoting our new product line and increasing follower interaction.Our URL is X”
- Create questions for lead generation, customer satisfaction surveys, and interviews: Formulate questions to gather valuable insights from leads, customers, or interviewees to inform decision-making processes.
- [Prompt example] “Formulate 15 questions for a customer satisfaction survey to gather insights on our new service and identify areas for improvement.”
- [Prompt example] “Create 5 interview questions to ask potential leads during initial sales calls to understand their needs and how our solutions can meet them.”
- Writing in your executive’s tone and style: Craft emails, memos, or other written communications in a way that reflects the executive’s tone.
- [Prompt example] “Compose a memo from the executive to the team, encouraging them after a successful project completion and outlining the next steps, maintaining the executive’s tone of voice.”
- [Prompt example] “Draft responses to comments on the CEO’s LinkedIn posts, ensuring the replies reflect the CEO’s professional and engaging tone. Here are 10 other previous responses she’s said as examples (attach screenshots).”
- [Prompt example] “Create a template for responding to congratulatory comments on the CEO’s LinkedIn profile, expressing gratitude and maintaining their funny and appreciative style.”
Concept clarification
- Understand difficult concepts: Seek explanations for or simplification of complex concepts or terms.
- [Prompt example] “Explain the concept of blockchain technology in simple terms suitable for someone with no technical background.”
- [Prompt example] “What are the most significant challenges facing the implementation of technology in healthcare, and how are companies addressing these issues?”
Optimizing productivity and workflow
- Enhance productivity: Seek advice on optimizing personal workflow and time management strategies.
- [Prompt example] “What are 5 ways to foster a collaborative environment as an executive assistant to a 20-person, Series A startup CEO based in the US?”
- Automate repetitive tasks: Explore automation tools or methods to streamline repetitive tasks and reduce manual workload.
- [Prompt example] “Explore methods to automate data entry tasks for our healthcare CRM system to save time and minimize errors.”
- Avoid getting stuck: Seek guidance on how to approach unfamiliar tasks or situations to overcome obstacles and achieve objectives.
- [Prompt example] “I’m an executive assistant, my executive just asked me to do {add task}. Where do I start?”
- [Prompt example] “Provide guidance on handling {an unfamiliar software tool} that I need to use for an upcoming project.”
Personal queries
- Personal questions: Seek information or clarification on various topics of personal interest or curiosity to broaden knowledge and understanding.
- [Prompt example] “What are wellness activities that I can add to my daily routine to avoid burnout?”
- [Prompt example] “Make a 5-day meal plan for busy people. Include plenty of vegetables and meat recipes only twice a week.”
AI’s proven impact on EA automation goes beyond the hype
The use of LLMs or AI in the workplace is nothing new and is clearly gaining traction. Think Grammarly, Calendly, Magical, and others. Executive assistants are leveraging all of this technology to better support the executives they work with. The fast-paced environment of startups seems to be calling for it.
Here are some examples of what we mean:
- EAs use Grammarly to proofread and improve the quality of emails, reports, and presentations. It’s great for catching typos and ensuring the writing is clear.
- Calendly allows users to determine specific time slots in their calendars for meetings. Stakeholders can quickly self-schedule an appointment. EAs can make sure that there are spots for customers always available and arrange meeting agendas whenever a new appointment is scheduled.
- EAs use Magical to automate repetitive tasks such as email templates, signatures, instructions, reminders, and greetings. Using a single command, they can easily retrieve these templates.
- Otter.ai is used to transcribe meetings and interviews. EAs use transcripts to extract action items, create workflows or processes, and document information. These transcripts are shared with the leadership team for further reference.
Concluding thoughts
In conclusion, the landscape of artificial intelligence is evolving and its usage in the workplace is increasingly common. Companies that are looking for fast growth can find automation tools greatly beneficial for their efficiency.
Executive assistants can be great allies not only in using AI tools, but also in implementing their usage across the organization. Having an executive assistant who is trained in these technologies is a contributing factor to success. Since technologies are ever-changing and evolving, an executive assistant also needs to be proactive and curious, with critical thinking and analytical skills.
These days, adopting extreme views on AI—whether idolizing it or fearing it—is not the most effective approach to new technologies. Instead, recognizing that AI tools are designed to complement human effort can lead to remarkable outcomes. We see the future as EAs + AI. Not EAs replaced by AI. If you’re curious to learn more about how we do that at Viva, let’s chat.
Tell us the skills you need and we'll find the best developer for you in days, not weeks.